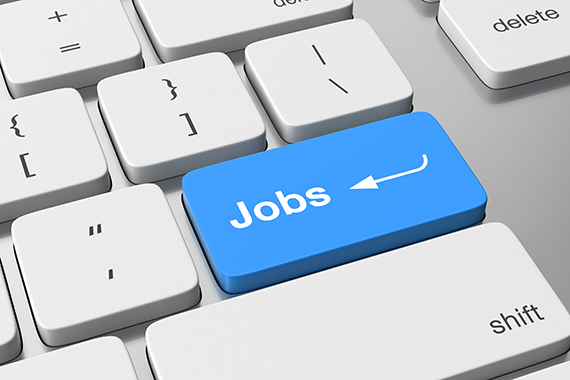
Scoring a Job
Krannert researcher finds that computers can be ‘trained’ to cost-effectively improve hiring practices
Millions of employment decisions are made each year, which can be a strategic advantage for those companies with the ability to sift through numerous candidates to find the best hire.
Michael A. Campion, Purdue’s Herman C. Krannert of Professor of Management, says the resumes and achievement records of most job seekers are part of an overflowing pool of big data that creates a daunting amount of work for employers and their HR staff, especially for companies with highly selective hiring practices.
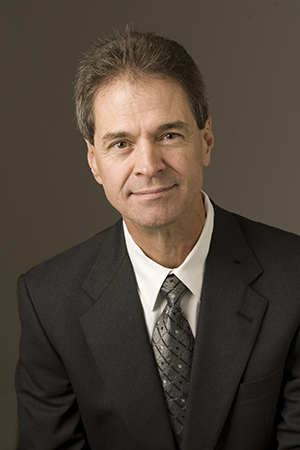
“Emerging advancements including the exponentially growing availability of computer-collected data and increasingly sophisticated statistical software have led organizations to begin using large-scale data analysis to improve their effectiveness,” Campion says. “Yet, little is known regarding how organizations can leverage these advancements to develop more effective personnel selection procedures, especially when the data are unstructured and text-based.”
Campion, who is listed as the second most cited author in human resource management textbooks in the Academy of Management journal Learning and Education, was among the first in his field to address the issue, co-authoring “Initial Investigation into Computer Scoring of Candidate Essays for Personnel Selection” for the Journal of Applied Psychology in 2016. It was co-authored by Michael C. Campion at the University of South Carolina, Emily D. Campion at the University of Buffalo SUNY, and Mathew H. Reider of Reider Research.
“Drawing on literature on natural language processing, we looked at the possibility of leveraging advances in text mining and predictive modeling computer software programs as a surrogate for human raters in the context of job selection,” Campion says. “Using records of nearly 46,000 job candidates in a large organization, we examine the validity of the scores to predict the ratings of panels of trained assessors, demonstrate that the practice does not disadvantage minority groups, and illustrate the positive financial impact of adopting it in an organization.”
The researchers also explain how to “train” a computer program to emulate a human rater when scoring employment records, which are defined as narrative descriptions candidates provide of past accomplishments like written answers to common interview questions.
Campion and his colleagues began by extracting up to 5,000 features or “concepts.” The researcher trains the computer by combining, eliminating and revising them to narrow the concepts to about 1,500 categories. Regression analysis is then used to select categories showing statistically significant criteria that aligns with human rater scores.
Campion says the study’s purpose was to alert scholars and practitioners to the potential advantages and disadvantages associated with using text mining and predictive computer modeling as an alternative to human raters in a selection context. In doing so, it also produced a bottom-line finding that offers additional appeal to companies using or considering the process.
“It appears possible that computer scoring can result in substantial cost savings when used to augment other information on job applicants,” Campion says. “Most directly, these techniques could enable the use of selection procedures for large-scale application that were previously too expensive. For example, these techniques could be used to inexpensively process and score the often large number of applications organizations receive. In the organization used in this research, the algorithm saves $200,000 per year in assessor time.
“Presently, applications are generally scored using rudimentary information retrieval programs such as keyword searches. Computer scoring may not only provide a more cost-effective alternative to separating those who are qualified from those you are not, but may also identify applicants that would otherwise not have been recognized as qualified due to their use of terms other than the keywords to describe their skills.”
The study also indicates that computer scoring is fairer. “Unlike humans, it does not have any bias because it consistently applies criteria across candidates,” Campion says.